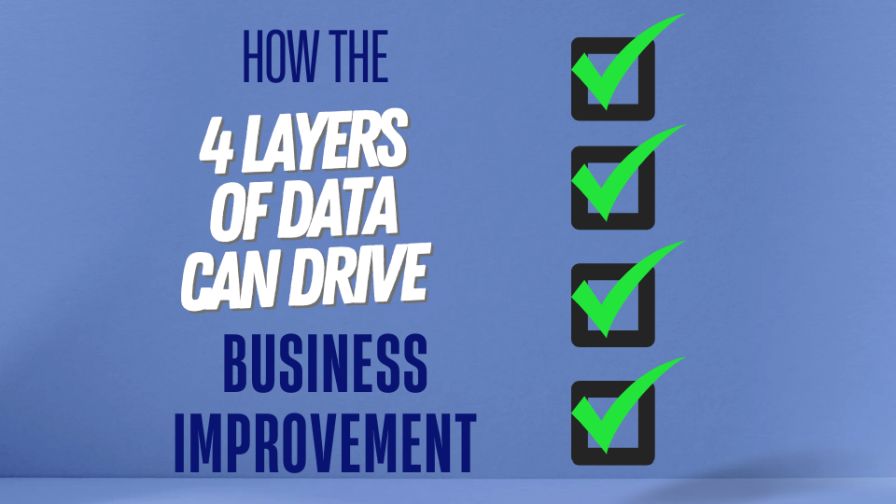
How the 4 Layers of Data Can Drive Business Improvement | Advanced Grower Solutions
Modern grower nursery and greenhouse business operations require analytics to help drive improvements.
There are several aspects to an effective analytics program, that can be viewed as layers or categories of functionality. Each higher layer depends on the lower layers. Some of the layers are part of a normal greenhouse software or nursery inventory management system. The higher layers, and thus more computationally complex, are sometimes done in other tools and environments.
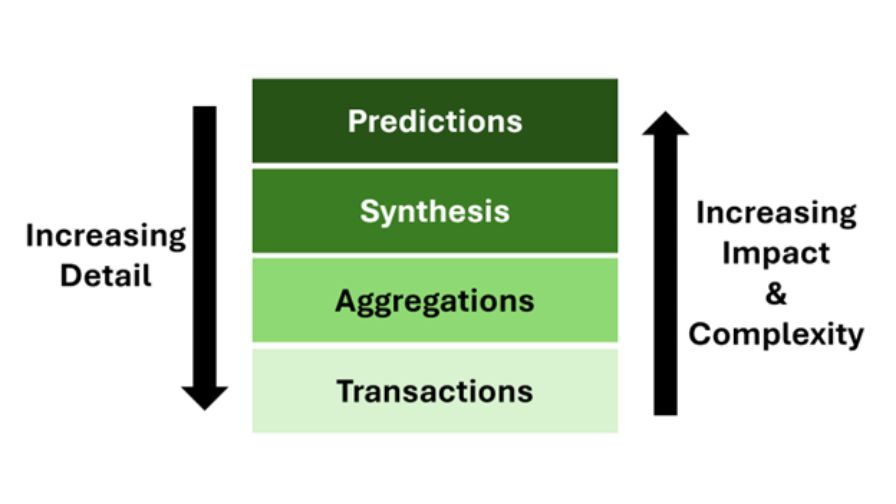
Layers of an effective analytics program | Advanced Grower Solutions
1. Transactions
At the lowest, most fundamental level of your data program is transactions. Usually, this is done by your greenhouse or nursery inventory software system.
Transactions are the individual ‘events’ that occur in your business. Transaction events mark the occurrence of something important that your business needs to track. For growers, these events could include:
- Transplanting or producing an item the potting line
- Receiving some buy-in material
- An inventory size bump up
- An inventory move or space action
- A sales quote or order, a pick or pull slip
- A dig
- A dump or shrink record
- An invoice or credit
- A customer payment
Transactions are the little actions taken every day that record the activity of your business and can be used to form the basis for your corporate financials and operational metrics you use to drive improvements. Properly recording transactions also drives more accurate availability, an important sales and customer relationship tool.
2. Aggregations
The next layer of a beneficial data program is the aggregations. Aggregations are mathematical calculations that involve data from multiple transactions.
For a very simple example, summing all the item sales records for a given week can yield gross item revenue and quantities shipped for a given week. The total for quantity and for revenue is a simple aggregation – literally aggregating, or mathematically combining (in this case a summation), across many records to get an individual answer. In this simple example, we aggregated all transactions across a given date range:
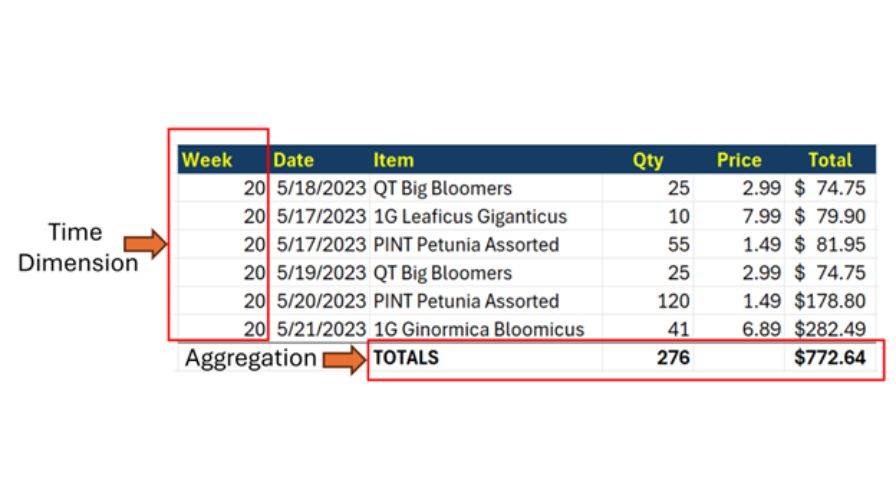
Data aggregation example | Advanced Grower Solutions
Dates are one important dimension by which your data can be aggregated. Other typical grower data dimensions for aggregations are things such as Customers, Customer Types, Items, Item Categories, or Sizes. Further, aggregations can be done across multiple dimensions, typically combining a Date or Time dimension with one of the others like plant category to yield revenue by plant category by week for example.
A grower’s financial statements are aggregations across a given month or year for defined financial categories. There are many calculated operational metrics a grower needs that are calculated across these dimensions, such as labor $ per hour or unit or average price for item categories by customer type.
3. Synthesis
The third layer of an effective data analytics program is synthesis. In this context ‘synthesis’ means combining different data sets to yield new insights from your data.
Synthesis takes different but related data sets, brings them together, and then performs calculations, aggregation, and analytics on them. It requires understanding how the different data sets can be linked accurately and then used for synthetic metrics.
An example given below is linking payroll data with production line data to yield labor rate metrics for the costs of production line activities:
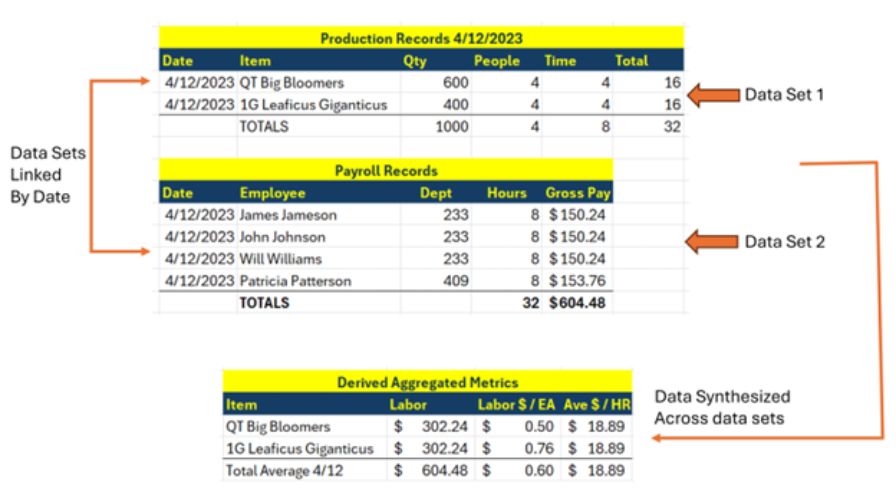
Data synthesis examples across data sets | Advanced Grower Solutions
4. Prediction
The fourth layer of an effective data analytics program is prediction. Prediction takes the relevant data from your history and current situation with stated knowns and assumptions and calculates some future value.
For example, a production budget is a prediction derived from your existing data, your production plan, your product metadata, and your labor metrics and raw materials costs.
For additional information and an expanded look at the aforementioned data layers and insights they can bring to your greenhouse or nursery business, make sure to read the original article on the Advanced Grower Solutions website.
0
1
5
4 Data Layers That Propel Business Growth and Improvement